作者:英特爾邊緣計算創(chuàng)新大使 盧雨畋
01概述
本文介紹了在英特爾 13 代酷睿 CPU i5 - 13490F 設(shè)備上部署 Qwen 1.8B 模型的過程,你需要至少 16GB 內(nèi)存的機器來完成這項任務(wù),我們將使用英特爾的大模型推理庫 [BigDL] 來實現(xiàn)完整過程。
英特爾的大模型推理庫 [BigDL]:
BigDL-llm 是一個在英特爾設(shè)備上運行 LLM(大語言模型)的加速庫,通過 INT4/FP4/INT8/FP8 精度量化和架構(gòu)針對性優(yōu)化以實現(xiàn)大模型在英特爾 CPU、GPU 上的低資源占用與高速推理能力(適用于任何 PyTorch 模型)。
本文演示為了通用性,只涉及 CPU 相關(guān)的代碼,如果你想學(xué)習(xí)如何在英特爾 GPU 上部署大模型。
02環(huán)境配置
在開始之前,我們需要準(zhǔn)備好 bigdl-llm 以及之后部署的相關(guān)運行環(huán)境,我們推薦你在 python 3.9 的環(huán)境中進行之后的操作。
如果你發(fā)現(xiàn)下載速度過慢,可以嘗試更換默認(rèn)鏡像源:
pip config set global.index-url https://pypi.doubanio.com/simple`
%pip install --pre --upgrade bigdl-llm[all] %pip install gradio %pip install hf-transfer %pip install transformers_stream_generator einops %pip install tiktoken
左滑查看更多
03模型下載
首先,我們通過 huggingface-cli 獲取 qwen-1.8B 模型,耗時較長需要稍作等待;這里增加了環(huán)境變量,使用鏡像源進行下載加速。
import os # 設(shè)置環(huán)境變量 os.environ['HF_ENDPOINT'] = 'https://hf-mirror.com' # 下載模型 os.system('huggingface-cli download --resume-download qwen/Qwen-1_8B-Chat --local-dir qwen18chat_src')
左滑查看更多
04保存量化模型
為了實現(xiàn)大語言模型的低資源消耗推理,我們首先需要把模型量化到 int4 精度,隨后序列化保存在本地的相應(yīng)文件夾方便重復(fù)加載推理;利用 `save_low_bit` api 我們可以很容易實現(xiàn)這一步。
from bigdl.llm.transformers import AutoModelForCausalLM from transformers import AutoTokenizer import os if __name__ == '__main__': model_path = os.path.join(os.getcwd(),"qwen18chat_src") model = AutoModelForCausalLM.from_pretrained(model_path, load_in_low_bit='sym_int4', trust_remote_code=True) tokenizer = AutoTokenizer.from_pretrained(model_path, trust_remote_code=True) model.save_low_bit('qwen18chat_int4') tokenizer.save_pretrained('qwen18chat_int4')
左滑查看更多
05加載量化模型
保存 int4 模型文件后,我們便可以把他加載到內(nèi)存進行進一步推理;如果你在本機上無法導(dǎo)出量化模型,也可以在更大內(nèi)存的機器中保存模型再轉(zhuǎn)移到小內(nèi)存的端側(cè)設(shè)備中運行,大部分常用家用 PC 即可滿足 int4 模型實際運行的資源需求。
import torch import time from bigdl.llm.transformers import AutoModelForCausalLM from transformers import AutoTokenizer QWEN_PROMPT_FORMAT = "{prompt} " load_path = "qwen18chat_int4" model = AutoModelForCausalLM.load_low_bit(load_path, trust_remote_code=True) tokenizer = AutoTokenizer.from_pretrained(load_path, trust_remote_code=True) input_str = "給我講一個年輕人奮斗創(chuàng)業(yè)最終取得成功的故事" with torch.inference_mode(): prompt = QWEN_PROMPT_FORMAT.format(prompt=input_str) input_ids = tokenizer.encode(prompt, return_tensors="pt") st = time.time() output = model.generate(input_ids, max_new_tokens=512) end = time.time() output_str = tokenizer.decode(output[0], skip_special_tokens=True) print(f'Inference time: {end-st} s') print('-'*20, 'Prompt', '-'*20) print(prompt) print('-'*20, 'Output', '-'*20) print(output_str)
左滑查看更多
06gradio-demo 體驗
為了得到更好的多輪對話體驗,這里還提供了一個簡單的 `gradio` demo界面方便調(diào)試使用,你可以修改內(nèi)置 `system` 信息甚至微調(diào)模型讓本地模型更接近你設(shè)想中的大模型需求。
import gradio as gr import time from bigdl.llm.transformers import AutoModelForCausalLM from transformers import AutoTokenizer QWEN_PROMPT_FORMAT = "{prompt} " load_path = "qwen18chat_int4" model = AutoModelForCausalLM.load_low_bit(load_path, trust_remote_code=True) tokenizer = AutoTokenizer.from_pretrained(load_path,trust_remote_code=True) def add_text(history, text): _, history = model.chat(tokenizer, text, history=history) return history, gr.Textbox(value="", interactive=False) def bot(history): response = history[-1][1] history[-1][1] = "" for character in response: history[-1][1] += character time.sleep(0.05) yield history with gr.Blocks() as demo: chatbot = gr.Chatbot( [], elem_id="chatbot", bubble_full_width=False, ) with gr.Row(): txt = gr.Textbox( scale=4, show_label=False, placeholder="Enter text and press enter", container=False, ) txt_msg = txt.submit(add_text, [chatbot, txt], [chatbot, txt], queue=False).then( bot, chatbot, chatbot, api_name="bot_response" ) txt_msg.then(lambda: gr.Textbox(interactive=True), None, [txt], queue=False) demo.queue() demo.launch()
左滑查看更多
利用英特爾的大語言模型推理框架,我們可以實現(xiàn)大模型在英特爾端側(cè)設(shè)備的高性能推理。只需要 2G 內(nèi)存占用就可以實現(xiàn)與本地大模型的流暢對話,一起來體驗下吧。
審核編輯:湯梓紅
-
英特爾
+關(guān)注
關(guān)注
61文章
10149瀏覽量
173711 -
cpu
+關(guān)注
關(guān)注
68文章
11015瀏覽量
215425 -
模型
+關(guān)注
關(guān)注
1文章
3471瀏覽量
49874
原文標(biāo)題:英特爾 CPU 實戰(zhàn)部署阿里大語言模型千問 Qwen-1_8B-chat | 開發(fā)者實戰(zhàn)
文章出處:【微信號:英特爾物聯(lián)網(wǎng),微信公眾號:英特爾物聯(lián)網(wǎng)】歡迎添加關(guān)注!文章轉(zhuǎn)載請注明出處。
發(fā)布評論請先 登錄
Intel OpenVINO? Day0 實現(xiàn)阿里通義 Qwen3 快速部署
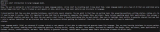
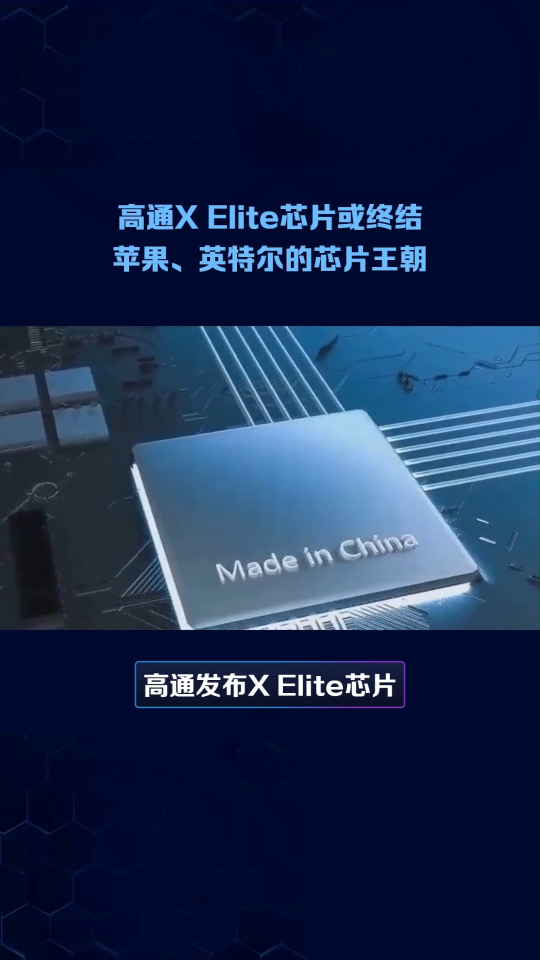
英特爾多款平板電腦CPU將于明年推出
啟用英特爾Optane被視為“1.8TB硬盤+英特爾Optane”是什么原因?
英特爾愛迪生閃存失敗
為什么選擇加入英特爾?
英特爾重點發(fā)布o(jì)neAPI v1.0,異構(gòu)編程器到底是什么
介紹英特爾?分布式OpenVINO?工具包
英特爾重新思考解決芯片短缺的常用基板
英特爾移動CPU
英特爾cpu爆驚天漏洞_英特爾cpu漏洞檢測_英特爾cpu檢測工具有哪些
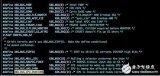
評論